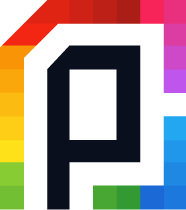
Delve further into the world of data analytics for claims professionals with the other articles in our monthly editorial series. Each piece focuses on a different aspect of the data-driven revolution, from predictive analytics to AI technologies. Enhance your skills and stay ahead of the curve by discovering new trends and best practices. Don't miss the chance to expand your knowledge and improve your claims management expertise by exploring the rest of this enlightening series.