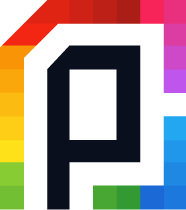
Insurance fraud is becoming increasingly complex and harder to detect through traditional methods alone. As fraud schemes evolve, claims adjusters need advanced tools to stay ahead of fraudulent activity. Predictive analytics, a powerful form of data analysis, allows adjusters to anticipate fraud patterns before they escalate. This article explores how predictive analytics can be applied to fraud detection in insurance claims, helping adjusters to identify high-risk cases early and take preventative action.
Predictive analytics involves using historical data to forecast future outcomes. In fraud detection, predictive models analyze past claims data, uncovering trends and identifying characteristics associated with fraudulent behavior. By leveraging these insights, adjusters can prioritize claims with a higher likelihood of fraud, ensuring these cases receive closer examination.
Predictive analytics uses several key techniques to detect fraud:
These techniques help adjusters assess the fraud risk of each claim, allowing them to focus resources on the cases most likely to involve fraudulent activity.
Integrating predictive analytics into the claims process brings several benefits for fraud detection. By using data-driven insights, adjusters can improve fraud detection accuracy, reduce costs associated with fraudulent payouts, and increase overall efficiency in claims processing. Here are some of the main benefits:
Overall, predictive analytics provides adjusters with a proactive approach to fraud detection, giving them the insights needed to address fraud before it escalates.
Developing a predictive model for fraud detection involves several steps. While larger insurance companies may have dedicated data science teams, smaller organizations can also adopt predictive analytics by partnering with third-party providers or utilizing off-the-shelf solutions. Heres a general approach to building a predictive model:
The first step is gathering historical claims data, which includes information about claims identified as fraudulent and legitimate. Data must be cleaned and standardized to ensure consistent formatting, which improves the accuracy of the predictive model.
Next, select the variables that are most likely to indicate fraud. These may include factors such as claim amount, type of damage, claimant history, and timing of the claim. Selecting relevant variables helps the model focus on the most critical information for fraud detection.
Using machine learning algorithms, train the model with the historical data. This process allows the model to learn from past claims and identify patterns associated with fraud. Training is essential to creating an accurate and effective model.
After training, validate the model by testing it on a new set of data to assess its accuracy. By comparing predictions to actual outcomes, adjusters can determine whether the model accurately identifies fraud. Adjustments may be necessary to improve accuracy.
Once trained, the predictive model can be deployed within the claims process, providing adjusters with real-time fraud risk scores for each claim.
Several insurance companies have successfully integrated predictive analytics into their fraud detection processes, achieving notable results. Here are some examples of real-life applications:
These real-life applications demonstrate how predictive analytics enables insurance companies to combat fraud more effectively, protecting both their bottom line and their reputation.
To incorporate predictive analytics into the claims process, insurers and adjusters should follow a strategic approach. Here are some key steps to ensure successful implementation:
By following these steps, insurers can integrate predictive analytics into their operations effectively, enhancing fraud detection capabilities across the organization.
Despite its advantages, implementing predictive analytics for fraud detection presents challenges. Common hurdles include data quality, integration with existing systems, and resistance to adopting new technologies. Addressing these challenges is essential for a successful transition.
To overcome these issues, insurers can:
Addressing these challenges helps organizations realize the full potential of predictive analytics in fraud detection.
As technology advances, predictive analytics will play an even more significant role in fraud detection. Innovations in machine learning and big data analytics are expected to increase the accuracy and speed of fraud detection, allowing adjusters to stay one step ahead of fraudsters.
In the near future, we may see:
These advancements promise to make predictive analytics an essential tool for adjusters in their ongoing fight against fraud.
Fraud detection is a critical aspect of the claims process, and predictive analytics offers a proactive approach to identifying and preventing fraudulent activity. By leveraging data-driven insights, adjusters can make informed decisions, prioritize high-risk claims, and ultimately protect their organizations from financial losses.
As predictive analytics technology evolves, its impact on fraud detection will only grow. Adjusters who embrace these tools will be better equipped to handle the challenges of modern claims processing, staying one step ahead of fraudsters and safeguarding their organizations.
Join us as we delve into these transformative topics, offering actionable advice and insights that claims adjusters can put into practice. This series, "Integrating Cutting-Edge Tech to Revolutionize Claims Processing," will equip you with the knowledge to confidently embrace technological change in your role. From identifying the most impactful tools to understanding best practices, our editorial series provides a comprehensive look at how tech innovations are reshaping claims work.
As the industry embraces digital transformation, it’s crucial for claims adjusters to stay ahead. Our series, "Integrating Cutting-Edge Tech to Revolutionize Claims Processing," delivers practical guidance on the latest technology trends, giving you a roadmap to excel in a tech-forward claims environment.